
In brief |
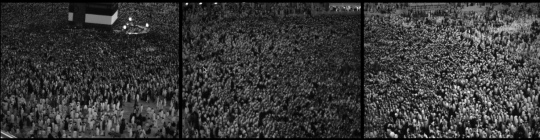
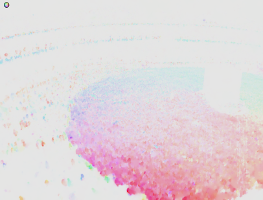
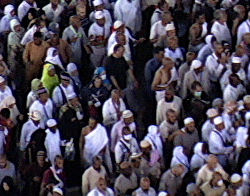
One of the most active recent developments in computer vision has been the analysis of crowded scenes. The interest that this specific field has raised may be explained from two different perspectives. In terms of applicability, continuous surveillance of public and sensitive areas has benefited from the advancements in hardware and infrastructure, and the bottleneck moved towards the processing level, where human supervision is a laborious task which often requires experienced operators. Other circumstances involving the analysis of dense crowds are represented by large scale events (sport events, religious or social gatherings) which are characterized by very high densities (at least locally) and an increased risk of congestions. From a scientific perspective, the detection of pedestrians in different circumstances, and fur- thermore the interpretation of their actions involve a wide range of branches of computer vision and machine learning.
Single camera analysis This represents the typical setup for a broad range of applications related to prevention and detection in public and private environments. Although some cam- era networks may contain thousands of units, it is quite common to perform processing tasks separately in each view. However, single view analysis is limited by the field of view of indi- vidual cameras and furthermore by the spatial layout of the scene; also, frequent occlusions in crowded scenes hamper the performance of standard detection algorithms and complexify tracking.
Multiple camera analysis Multiple camera analysis has the potential to overcome problems related to occluded scenes, long trajectory tracking or coverage of wider areas. Among the main scientific challenges, these systems require mapping different views to the same coordi- nate system; also, solutions for the novel problems they address (detection in dense crowds, object and track association, re-identification etc.) may not be obtained simply by employing and extending previous strategies used in single camera analysis.
In our study, we focus on solving the problem of analyzing the dynamics of a high-density crowd. The goal of the present proposal is to tackle the major challenge of detecting and tracking simultaneously as particles thousands of pedestrians forming a high-density crowd, and based on real data observations, to assist in proposing and validating a particle interaction model for crowd flow. Our project is original in its aim of performing particle level analysis, as well as through its emphasis on wide area multiple camera tracking. The strategy we intend to follow is based on a feedback loop involving particle segmentation and tracking, which aims to address the main difficulty of this problem, the uncertainty of data association. The value of such a study rests on the need for better solutions for human urban environments and for transport infrastructures, that not only improve the efficiency of the flows involved, but also do it in such a way as to increase and not diminish the quality of life. Another important prerogative of such research is to prevent fatalities during large scale events and gatherings.
Main objectives Toward the end of the project, we intend to propose a methodology for the analysis of high-density crowds which benefits from the recent developments in single camera tracking, and also proposes effective data association solutions among multiple cameras. Secondly, we intend to support the research community by providing a multi-camera dataset which would also allow for a stronger implication of additional fields involved in the general study of crowds, mainly physics, control, simulations and sociology.
People involved |
Researchers:
- Emanuel Aldea: project coordinator
- Sylvie Le Hégarat-Mascle: collaborator, image processing
- Séverine Dubuisson: collaborator, tracking
- Khurom Kiyani: collaborator, physical models and analysis
- Gianni Franchi: postdoc
- Jennifer Vandoni: PhD student
- Nicola Pellicanò: PhD student
- Kevin Mercier: Master intern
- Alireza Rezaei: Master intern
- Camille Palmier: Master intern
- Raphaël Guegan: Master intern
- Huiqin Chen: Master intern
- Davide Marastoni: Master intern
Papers published or submitted for publication |
@article{franchi19arxiv, title={TRADI: Tracking deep neural network weight distributions}, author={Franchi, Gianni and Bursuc, Andrei and Aldea, Emanuel and Dubuisson, S{\'e}verine and Bloch, Isabelle}, journal={arXiv preprint arXiv:1912.11316}, year={2019} }
@inproceedings{aldea19avss, title = { Constraining Relative Camera Pose Estimation with Pedestrian Detector-Based Correspondence Filters}, author = {Aldea, Emanuel and Pollok, Thomas and Qu, Chengchao}, booktitle = {Proceedings of the 16th IEEE International Conference on Advanced Video and Signal based Surveillance (AVSS)}, year = {2019} }
@inproceedings{franchi19avss, title = {Crowd Behaviour Characterization for Scene Tracking}, author = {Franchi, Gianni and Aldea, Emanuel and Dubuisson, S{\'e}verine and Bloch, Isabelle}, booktitle = {Proceedings of the 16th IEEE International Conference on Advanced Video and Signal based Surveillance (AVSS)}, year = {2019} }
@Article{Pellicano2019, author="Pellican{\`o}, Nicola and Aldea, Emanuel and Le H{\'e}garat-Mascle, Sylvie", title="Wide baseline pose estimation from video with a density-based uncertainty model", journal="Machine Vision and Applications", year="2019", month="Jun", day="13", abstract="Robust wide baseline pose estimation is an essential step in the deployment of smart camera networks. In this work, we highlight some current limitations of conventional strategies for relative pose estimation in difficult urban scenes. Then, we propose a solution which relies on an adaptive search of corresponding interest points in synchronized video streams which allows us to converge robustly toward a high-quality solution. The core idea of our algorithm is to build across the image space a nonstationary mapping of the local pose estimation uncertainty, based on the spatial distribution of interest points. Subsequently, the mapping guides the selection of new observations from the video stream in order to prioritize the coverage of areas of high uncertainty. With an additional step in the initial stage, the proposed algorithm may also be used for refining an existing pose estimation based on the video data; this mode allows for performing a data-driven self-calibration task for stereo rigs for which accuracy is critical, such as onboard medical or vehicular systems. We validate our method on three different datasets which cover typical scenarios in pose estimation. The results show a fast and robust convergence of the solution, with a significant improvement, compared to single image-based alternatives, of the RMSE of ground-truth matches, and of the maximum absolute error.", issn="1432-1769", doi="10.1007/s00138-019-01036-6", url="https://doi.org/10.1007/s00138-019-01036-6" }
@inproceedings{vandoni19icip, author = {Vandoni, Jennifer and Aldea, Emanuel and Le H{\'e}garat-Mascle, Sylvie }, booktitle = {Proceedings of the 26th International Conference on Image Processing (ICIP) }, title = {Evaluating Crowd Density Estimators via Their Uncertainty Bounds}, year = {2019} }
@inproceedings{vandoni19ijar, title = "Evidential query-by-committee active learning for pedestrian detection in high-density crowds", journal = "International Journal of Approximate Reasoning", volume = "104", pages = "166 - 184", year = "2019", issn = "0888-613X", doi = "https://doi.org/10.1016/j.ijar.2018.11.007", url = "http://www.sciencedirect.com/science/article/pii/S0888613X18303517", author = "Jennifer Vandoni and Emanuel Aldea and Sylvie Le Hégarat-Mascle" }
@inproceedings{rezaei18avss, author = {Rezaei, Alireza and Pellican{\`o}, Nicola and Aldea, Emanuel }, booktitle = {Proceedings of the 15th IEEE International Conference on Advanced Video and Signal based Surveillance (AVSS)}, title = {GPU-accelerated Height Map Estimation with Local Geometry Priors in Large Scenes}, year = {2018} }
@inproceedings{pellicano18ijar, author = {Pellican{\`o}, Nicola and Le H{\'e}garat-Mascle, Sylvie and Aldea, Emanuel }, title = "2CoBel: A scalable belief function representation for 2D discernment frames", journal = "International Journal of Approximate Reasoning", volume = "103", pages = "320 - 342", year = "2018", issn = "0888-613X", doi = "https://doi.org/10.1016/j.ijar.2018.10.007", url = "http://www.sciencedirect.com/science/article/pii/S0888613X18303864" }
@inproceedings{pellicano18fusion, author = {Pellican{\`o}, Nicola and Le H{\'e}garat-Mascle, Sylvie and Aldea, Emanuel }, booktitle = {Proceedings of the 21st International Conference on Information Fusion (FUSION)}, title = {2{C}o{B}el: An Efficient Belief Function Extension for Two-dimensional Continuous Spaces}, pages={1032-1039}, doi={10.23919/ICIF.2018.8455783}, year = {2018} }
@inproceedings{vandoni18fusion, author = {Vandoni, Jennifer and Le H{\'e}garat-Mascle, Sylvie and Aldea, Emanuel }, booktitle = {Proceedings of the 21st International Conference on Information Fusion (FUSION)}, title = {Belief Function Definition for Ensemble Methods - Application to Pedestrian Detection in Dense Crowds}, pages={2481-2488}, doi={10.23919/ICIF.2018.8455313}, year = {2018} }
@inproceedings{vandoni17avss, author = {Vandoni, Jennifer and Aldea, Emanuel and Le Hégarat-Mascle, Sylvie}, booktitle = {Proceedings of the 14th IEEE International Conference on Advanced Video and Signal based Surveillance (AVSS)}, title = {An Evidential Framework for Pedestrian Detection in High-Density Crowds}, year = {2017} }
@inproceedings{vandoni17spcrowd, author = {Vandoni, Jennifer and Aldea, Emanuel and Le Hégarat-Mascle, Sylvie}, booktitle = {Proceedings of the 14th IEEE International Conference on Advanced Video and Signal based Surveillance (AVSS) - 2nd Workshop on Signal Processing for Understanding Crowd Dynamics}, title = {Active Learning for High-Density Crowd Count Regression}, year = {2017} }
@inproceedings{pellicano17ammds, author = {Pellicanò, Nicola and Aldea, Emanuel and Le Hégarat-Mascle, Sylvie}, booktitle = {Proceedings of the 28th British Machine Vision Conference (BMVC) - 5th Activity Monitoring by Multiple Distributed Sensing Workshop}, title = {Geometry-Based Multiple Camera Head Detection in Dense Crowds}, year = {2017} }
@inproceedings{aldea17tgf, author = {Aldea, Emanuel and Kiyani, Khurom H.}, booktitle = {Proceedings of the 12th International Conference on Traffic and Granular Flow (TGF)}, title = {Pressure Estimation In A High-Density Crowd Using A Multi-Scale Count Regressor}, year = {2017} }
@inproceedings{pellicano16icpr, author = {Nicola Pellicano and Emanuel Aldea and Sylvie Le H{\'{e}}garat{-}Mascle}, title = {Robust wide baseline pose estimation from video}, booktitle = {23rd International Conference on Pattern Recognition, {ICPR} 2016, Canc{\'{u}}n, Mexico, December 4-8, 2016}, pages = {3820--3825}, year = {2016} }
Related code and data |
The files below support some of the publications. They are provided with a documentation and demo examples, and a short intro is also provided here.
Data
Part of the work is performed on a dataset containing three synchronized overlapping views of Regents Park Mosque sahn, featuring a sparse to medium density crowd. We also work on some high-density data recorded in Makkah. Depending on your needs (i.e. crowd density, low or high dynamics), an adapted sequence may be provided under the following agreement:
- strict academic research use
- no redistribution
Local entropy measures and crowd motion patterns
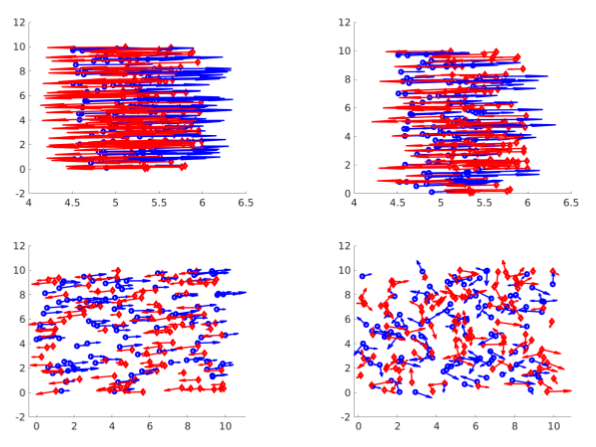
Code: soon available on GitHub
Data: annotations available as Crowd_annotation_Dataset.zip
In this work, we perform an in-depth analysis of the specific difficulties a crowded scene dataset raises for tracking algorithms. Starting from the standard characteristics depicting the crowd and their limitations, we introduce six entropy measures related to the motion patterns and to the appearance variability of the individuals forming the crowd, and one appearance measure based on Principal Component Analysis. The proposed measures are discussed on synthetic configurations and on multiple real datasets. For one of the real datasets, we performed additional annotations that are provided at the above link. Further details are provided in the following publication(s):
@inproceedings{franchi19avss, title = {Crowd Behaviour Characterization for Scene Tracking}, author = {Franchi, Gianni and Aldea, Emanuel and Dubuisson, S{\'e}verine and Bloch, Isabelle}, booktitle = {Proceedings of the 16th IEEE International Conference on Advanced Video and Signal based Surveillance (AVSS)}, year = {2019} }
Pose estimation from video

Code: available on GitHub
Data: a subset of the images we used in the evaluation is provided along with the code, in order to facilitate testing. If you would like to get the entire set, contact me.
The code allows you to refine the estimation of the relative pose between two synchronized cameras based on the video streams recorded by the cameras. For the two synchronized video streams (with people/cars/etc. moving around), the algorithm requires as input only the undistorted images. This algorithm might help you if:
- your scene is difficult (wide baseline, homogeneous areas, repetitive patterns), and a baseline pose estimation using an image pair fails consistently
- your scene does not allow for object based calibration
- object based calibration has been used, but you need better accuracy in some parts of the scene.

- to check the result of our algorithm (in the form of an error distribution in the image space, as in the image to the right)
- in an extreme case, to get a very accurate pose manually if nothing else works
@Article{Pellicano2019, author="Pellican{\`o}, Nicola and Aldea, Emanuel and Le H{\'e}garat-Mascle, Sylvie", title="Wide baseline pose estimation from video with a density-based uncertainty model", journal="Machine Vision and Applications", year="2019", month="Jun", day="13", abstract="Robust wide baseline pose estimation is an essential step in the deployment of smart camera networks. In this work, we highlight some current limitations of conventional strategies for relative pose estimation in difficult urban scenes. Then, we propose a solution which relies on an adaptive search of corresponding interest points in synchronized video streams which allows us to converge robustly toward a high-quality solution. The core idea of our algorithm is to build across the image space a nonstationary mapping of the local pose estimation uncertainty, based on the spatial distribution of interest points. Subsequently, the mapping guides the selection of new observations from the video stream in order to prioritize the coverage of areas of high uncertainty. With an additional step in the initial stage, the proposed algorithm may also be used for refining an existing pose estimation based on the video data; this mode allows for performing a data-driven self-calibration task for stereo rigs for which accuracy is critical, such as onboard medical or vehicular systems. We validate our method on three different datasets which cover typical scenarios in pose estimation. The results show a fast and robust convergence of the solution, with a significant improvement, compared to single image-based alternatives, of the RMSE of ground-truth matches, and of the maximum absolute error.", issn="1432-1769", doi="10.1007/s00138-019-01036-6", url="https://doi.org/10.1007/s00138-019-01036-6" }
@inproceedings{pellicano16icpr, author = {Pellican{\`o}, Nicola and Aldea, Emanuel and Le H{\'e}garat-Mascle, Sylvie }, booktitle = {Proceedings of the International Conference on Pattern Recognition (ICPR)}, title = {Robust Wide Baseline Pose Estimation from Video}, year = {2016} }
2CoBel: A Scalable Belief Function Representation for 2D Discernment Frames
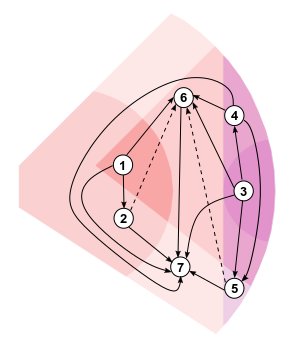
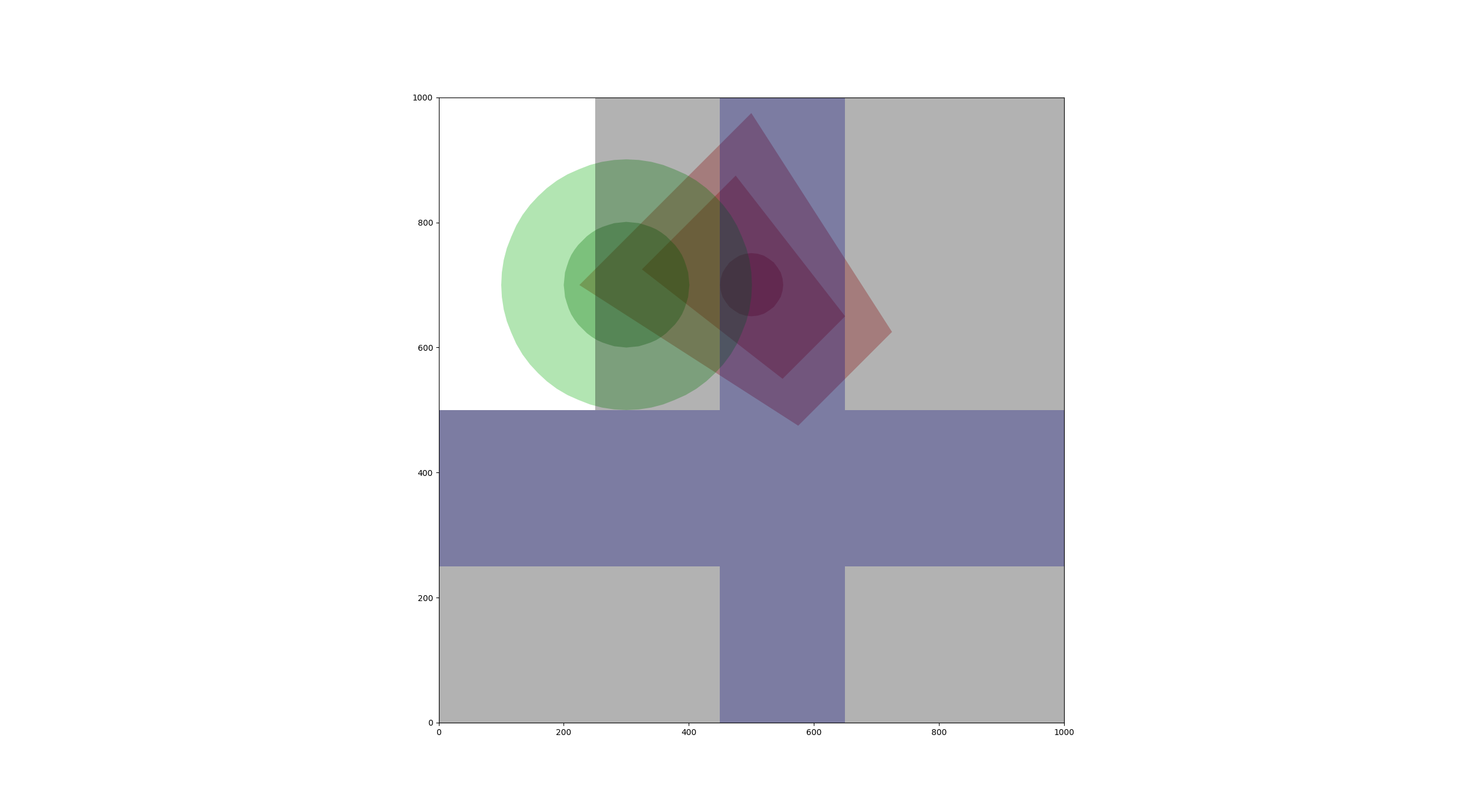
Code: available on GitHub
We introduce an innovative approach for handling 2D compound hypotheses within the Belief Function framework. We propose a polygon-based generic representation which relies on polygon clipping operators, as well as on a topological ordering of the focal elements within a directed acyclic graph encoding their interconnections. This approach allows us to make the computational cost for the hypothesis representation independent of the cardinality of the discernment frame. For BBA combination, canonical decomposition and decision making, we propose efficient algorithms which rely on hashes for fast lookup, and which benefit from the proposed graph representation. An implementation of the functionalities recalled above is provided as an open source library.
For MOHICANS, the library is currently used for multiple source pedestrian localization. We intend to develop a tracking algorithm for dense crowds, by performing cautious fusion of multiple detection sources provided by a smart camera network. We intend to highlight the interest of efficient 2D BFT representations for scaling such algorithms for high density crowds.
Further details are provided in the following publication(s):
@inproceedings{pellicano18ijar, author = {Pellican{\`o}, Nicola and Le H{\'e}garat-Mascle, Sylvie and Aldea, Emanuel }, title = "2CoBel: A scalable belief function representation for 2D discernment frames", journal = "International Journal of Approximate Reasoning", volume = "103", pages = "320 - 342", year = "2018", issn = "0888-613X", doi = "https://doi.org/10.1016/j.ijar.2018.10.007", url = "http://www.sciencedirect.com/science/article/pii/S0888613X18303864" }
@inproceedings{pellicano18fusion, author = {Pellicanò, Nicola and Le Hégarat-Mascle, Sylvie and Aldea, Emanuel }, booktitle = {Proceedings of the 21st International Conference on Information Fusion (FUSION)}, title = {2CoBel: An Efficient Belief Function Extension for Two-dimensional Continuous Spaces}, year = {2018} }